Unlocking the Future of Mobility: The Essential Role of Training Data for Self-Driving Cars
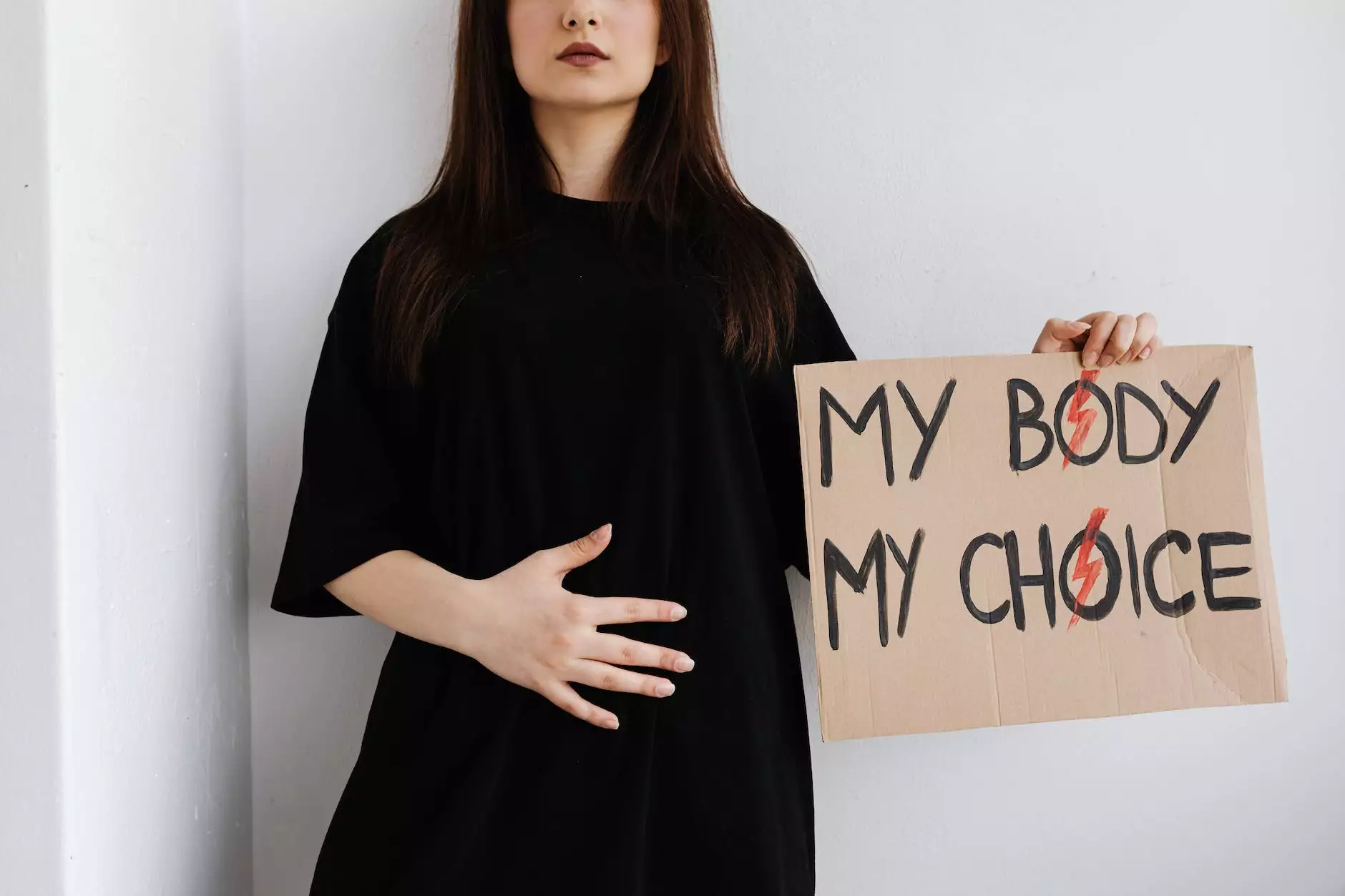
In the rapidly evolving landscape of technology, self-driving cars represent one of the most transformative innovations of our time. At the heart of these autonomous vehicles lies the training data for self-driving cars, which is crucial for their development and operational efficiency. In this extensive article, we will delve deep into the significance of training data, its collection, preparation, and the impact it has on the safety and performance of self-driving vehicles.
Understanding Self-Driving Technology
Self-driving technology, or autonomous driving, leverages advanced algorithms, machine learning models, and a plethora of sensors to navigate and operate vehicles without human intervention. With the ability to process vast amounts of data in real-time, self-driving cars can make instant decisions, drastically reducing the likelihood of human error, which is a leading cause of road accidents.
The Cornerstone: What is Training Data?
Training data refers to the large sets of information used to train machine learning algorithms. For self-driving cars, this data plays an integral role in teaching the vehicles how to interpret and respond to their surroundings. The quality, accuracy, and comprehensiveness of this data determine the effectiveness of the algorithms employed, highlighting its critical importance in the development of safe and reliable autonomous driving systems.
Types of Training Data for Self-Driving Cars
Training data can be classified into various types, each serving a distinct purpose in the learning and development processes of self-driving systems:
- Image Data: Visual data captured from cameras mounted on the vehicle provides essential information about the surrounding environment, including road signs, pedestrians, and other vehicles.
- Lidar Data: Light Detection and Ranging (Lidar) data provides three-dimensional representations of the environment, helping self-driving cars detect obstacles and gauge distances accurately.
- Radar Data: Radar systems complement camera and Lidar data by measuring the speed and distance of objects, crucial for adaptive cruise control and collision avoidance.
- GPS and Mapping Data: Precise geographic data helps self-driving cars navigate using pre-defined routes, ensuring that they can travel efficiently and safely.
- Behavioral Data: Data regarding typical driving behaviors and scenarios, such as how to navigate through roundabouts or manage lane changes, refine the learning process.
The Process of Collecting Training Data
Gathering training data for self-driving cars is a multifaceted process that involves several key steps:
1. Data Acquisition
Data acquisition involves collecting raw data from various sensors, including cameras, Lidar, and radar, during the operation of the vehicle in diverse environments and conditions. This raw data forms the foundational layer of the training sets.
2. Annotation
Once acquired, the data must be annotated accurately. This involves tagging images and videos to identify objects, road signs, barriers, and other critical elements within the data. Annotation is vital, as it provides context to the raw data, enabling algorithms to learn effectively.
3. Cleaning and Processing
Data cleaning and processing involve filtering out noise, correcting inaccuracies, and ensuring the quality of the data. High-quality training data is essential for developing algorithms that perform well under various scenarios.
4. Augmentation
Data augmentation techniques can be employed to artificially increase the size of the training dataset. This involves creating variations of existing data through techniques such as rotation, flipping, or adjusting brightness, which helps improve the model's robustness.
5. Validation and Testing
Before deployment, the training data must be validated and tested to ensure it meets the necessary quality standards. This includes using a separate validation dataset to evaluate the model’s performance and adjust accordingly.
The Importance of Diverse Training Data
Diversity in the training data is crucial for building effective self-driving systems. Models trained on a wide range of data can better generalize, leading to safer navigation in varying conditions and scenarios. A well-rounded dataset should include:
- Diverse Weather Conditions: Training data should encompass images and sensor readings taken during rain, snow, fog, and bright sunlight, enabling the algorithm to perform reliably regardless of environmental variations.
- Varietal Road Scenarios: Data should represent highway driving, urban navigation, rural roads, and intersections, capturing the complex dynamics of different driving environments.
- Population Density: Algorithms need exposure to densely populated areas with high pedestrian activity as well as low-density areas to adapt to varying levels of complexity in navigation.
- Unique Cultures and Traffic Rules: Including data from different regions allows self-driving cars to understand local traffic rules and cultural nuances in behavior.
Safety: The Primary Concern
The foremost priority of any self-driving technology is safety. Properly curated training data significantly enhances the reliability and predictability of autonomous vehicles. By simulating a plethora of scenarios during training, developers can anticipate potential challenges and fine-tune the decision-making processes of their vehicles. For instance, thorough training can help prevent accidents in complex urban settings by educating the system on how to interact responsibly with other road users.
Performance Optimization Through Continuous Learning
The field of self-driving cars is dynamic and continuously evolving. As more data is collected from real-world driving situations, algorithms can undergo ongoing training to improve performance. This concept, known as continuous learning, allows self-driving technologies to stay updated with emerging scenarios, enhancing their adaptability and efficiency over time.
Challenges in the Training Data Process
Despite the clear benefits, the journey of creating effective training data is fraught with challenges, including:
- Data Collection Costs: Acquiring high-quality training data can be resource-intensive and costly, requiring extensive hardware and software infrastructure.
- Legal and Ethical Concerns: Gathering data, especially related to human behavior, raises privacy and ethical questions that need to be addressed.
- Scalability: As self-driving technology advances, the need for larger and more diverse datasets increases, complicating the scalability of data collection efforts.
- Data Quality Assurance: Maintaining high standards for data quality across diverse datasets can be challenging, requiring ongoing monitoring and validation.
Keymakr: Pioneering in Software Development for Self-Driving Cars
Keymakr.com is at the forefront of revolutionizing software development for autonomous vehicles, focusing on the creation, management, and delivery of high-quality training data for self-driving cars. Our professional team combines advanced technology with a commitment to safety and innovation. We understand that by utilizing comprehensive and reliable training data, we can enhance the effectiveness of self-driving algorithms, ensuring they achieve the highest standards in safety and performance.
Why Choose Keymakr?
- Expertise: Our team comprises industry experts dedicated to delivering sophisticated software solutions tailored to the needs of self-driving car developers.
- Quality Assurance: We ensure that our training data meets the highest quality standards, providing a solid foundation for the development of robust autonomous vehicle systems.
- Diverse Data Solutions: Our capabilities range from image and Lidar data collection to behavior data analysis, offering a comprehensive suite of training data solutions.
- Adaptability: With our flexible approach, we can adjust and expand our data offerings to meet the evolving demands of the self-driving vehicle sector.
Conclusion: Shaping the Future with Quality Training Data
In conclusion, the importance of training data for self-driving cars cannot be overemphasized. It is the bedrock upon which the safety, efficiency, and reliability of autonomous vehicles are built. Ensuring access to diverse, high-quality, and well-annotated training data is essential for the continued advancement of self-driving technology. As we navigate the future of mobility, businesses like Keymakr play a pivotal role in shaping the landscape of autonomous vehicles through innovative software development solutions that harness the power of effective training data.
© 2023 Keymakr. All rights reserved.
training data for self driving cars