Effective Spam Detection Using Machine Learning in Modern Business
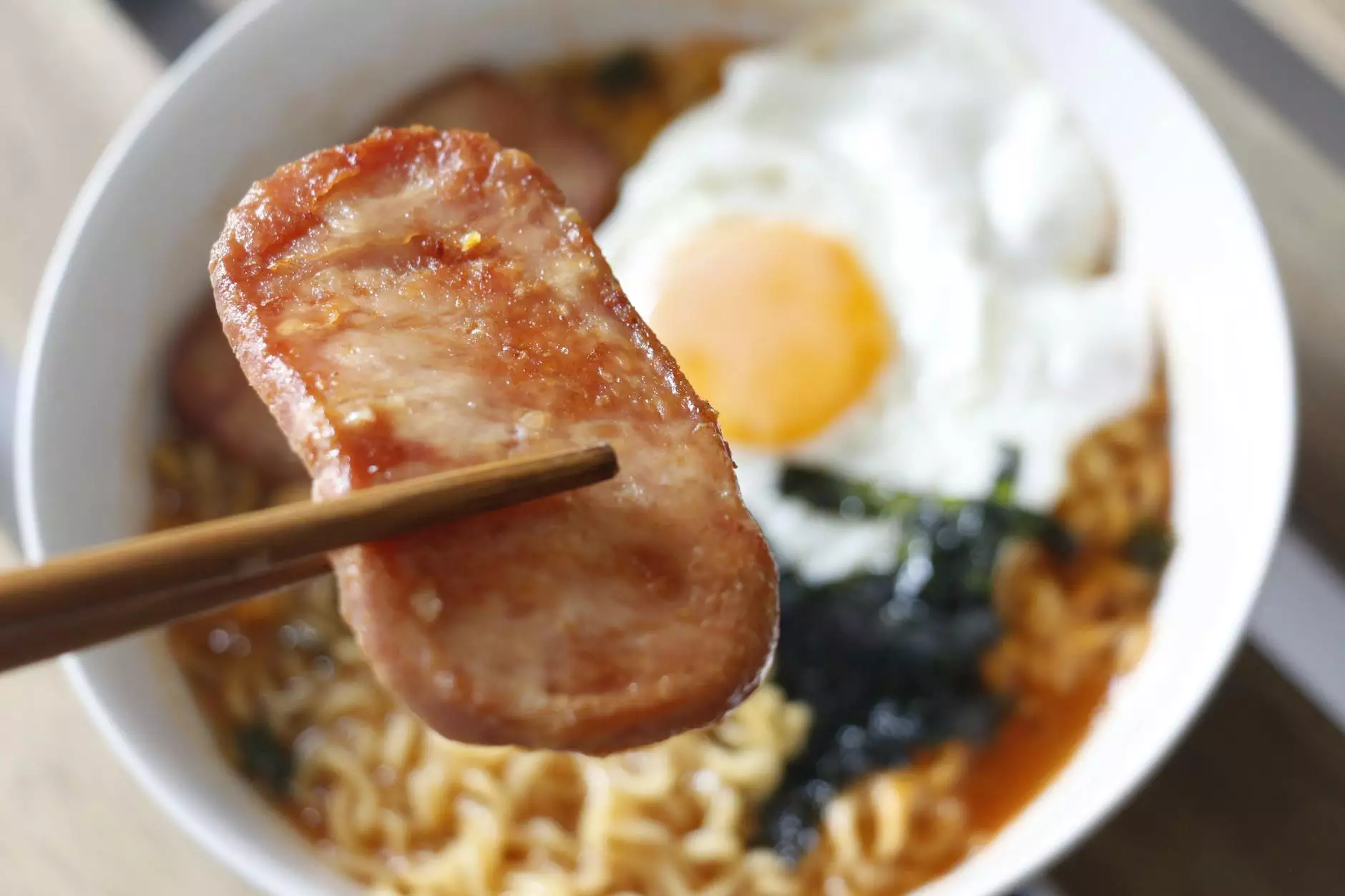
In today's digital landscape, businesses face an overwhelming amount of data and communication. With the rise of cyber threats, managing and filtering this influx of information is more important than ever. One of the most pressing issues is spam, which not only clutters inboxes but also poses severe security risks. This is where spam detection using machine learning comes into play. This comprehensive guide will explore the significance of machine learning in combating spam and outline how businesses can implement these innovative technologies to enhance their security systems.
Understanding Spam in the Digital Age
Spam refers to unsolicited messages, typically sent in bulk, often for malicious purposes such as phishing or spreading malware. According to recent statistics, approximately 45% of all emails sent are classified as spam. This staggering figure highlights the necessity for robust spam detection systems. As a part of the IT Services & Computer Repair sector, businesses must stay ahead of spam threats to protect their sensitive information and maintain operational efficiency.
What is Machine Learning?
Machine learning (ML) is a subset of artificial intelligence (AI) that enables systems to learn and improve from experience without being explicitly programmed. By analyzing vast amounts of data, ML algorithms can identify patterns, make decisions, and predict outcomes based on historical information. In terms of spam detection, machine learning can evolve and refine its techniques to outsmart even the most sophisticated spam tactics.
The Role of Machine Learning in Spam Detection
Integrating machine learning into spam detection systems allows businesses to improve their email filtering processes significantly. Here are some of the key benefits:
- Accuracy: ML algorithms can be trained on extensive datasets to distinguish between legitimate emails and spam with high precision.
- Real-Time Analysis: Machine learning models can continuously analyze incoming data, enabling real-time spam detection.
- Adaptability: By learning from new data, ML models can adapt to emerging spam techniques, ensuring ongoing effectiveness.
- Reduced False Positives: Machine learning helps in minimizing the number of misclassified emails, thus protecting important communications.
How Spam Detection Using Machine Learning Works
The process typically involves the following steps:
1. Data Collection
To train machine learning models, businesses first need to collect historical email data containing both spam and legitimate messages. This dataset serves as the backbone of the detection system.
2. Preprocessing the Data
The next step involves cleaning the email data. This includes removing duplicates, irrelevant information, and normalizing the text to ensure consistency. Techniques such as tokenization and stemming are often employed to transform the text into a format suitable for analysis.
3. Feature Extraction
In this phase, important features that differentiate spam from legitimate emails are identified. Common features include:
- Subject Line: Analysis of commonly used phrases in spam.
- Email Header: Examination of sending domains and IP addresses.
- Content Length: Lengthy messages are often regarded as spam.
- Links and Attachments: Spam often includes a higher number of links or attachments.
4. Choosing the Right Machine Learning Algorithm
Various algorithms can be utilized in spam detection, including:
- Naive Bayes Classifier: A popular choice for text classification tasks due to its simplicity and effectiveness.
- Support Vector Machines (SVM): This algorithm works well for larger datasets and provides high accuracy.
- Random Forest: This ensemble method reduces the risk of overfitting and improves the overall model performance.
- Deep Learning: Advanced techniques using neural networks can capture complex patterns within the data.
5. Training the Model
Once the appropriate algorithm is chosen, the next step is to train the model using the preprocessed data and extracted features. The model learns to classify emails based on the input data, identifying patterns that correlate with spam and legitimate messages.
6. Model Evaluation
To assess the effectiveness of the model, it is tested against a separate validation dataset. Key metrics such as accuracy, precision, recall, and F1 score are computed to evaluate performance. Adjustments may be made based on the results to enhance its ability to detect spam.
7. Integration and Deployment
After successfully building and evaluating the model, businesses can integrate it into their email systems. Continuous monitoring and updating of the model are essential to adapt to new spam techniques. Regular retraining with fresh data helps maintain accuracy over time.
Benefits of Spam Detection Using Machine Learning for Businesses
Implementing machine learning for spam detection provides numerous advantages to businesses:
- Enhanced Security: Protects sensitive information from phishing attacks and data breaches.
- Increased Productivity: Reduces time spent sorting through spam, allowing employees to focus on core business activities.
- Cost Savings: Automating the spam detection process minimizes the need for extensive manual intervention.
- Improved Customer Trust: By safeguarding communications, businesses foster better relationships with clients and partners.
Challenges in Spam Detection Using Machine Learning
Despite its effectiveness, there are several challenges businesses may encounter when implementing spam detection:
- High Volume of Data: Processing large datasets requires substantial computational resources.
- Evolving Spam Techniques: Spammers continuously adapt their strategies, necessitating frequent updates to detection models.
- Data Privacy Concerns: Handling sensitive data mandates compliance with regulations such as GDPR.
Conclusion
In a world inundated with digital communication, spam detection using machine learning stands out as a vital tool for businesses aiming to enhance their security frameworks. By leveraging advanced algorithms, organizations can protect themselves from the detrimental impacts of spam while optimizing their operations. Machine learning is not merely a trend; it represents the future of IT services and computer repair solutions. By investing in this technology, businesses can safeguard their data and maintain a competitive edge in their industry. Embrace the power of machine learning today to transform your spam detection processes and robustly defend your business against evolving threats.
Join the Revolution at Spambrella
At Spambrella, we specialize in implementing cutting-edge machine learning techniques for spam detection, tailored specifically for businesses in various industries. Our dedicated team of experts is committed to ensuring your organization stays protected from spam threats while streamlining your IT operations. Contact us today to learn more about our IT services and security systems designed to secure your business's future.